As a healthcare executive, you know data science is revolutionizing your industry. But do you grasp its full potential? This blog explores 10 common use cases that demonstrate the transformative power of data science in healthcare manufacturing. Whether improving production quality or predicting patient outcomes, data science enhances decision-making and unlocks innovation.
Join us as we delve into real-world examples, illustrating data science’s expanding role. From personalized medicine to optimizing clinical trials, you’ll discover how leading organizations leverage data to raise the bar for efficiency, accuracy, and patient care.
Let these insights spark ideas on how data science can help your organization achieve its mission.
Use Case 1: Predictive Maintenance
Predictive maintenance utilizes data science techniques like machine learning to analyze equipment data and predict when industrial assets are likely to fail. This allows healthcare manufacturers to:
Analyzing Sensor Data
Sensors within medical devices track factors like vibration, temperature, and pressure. Data scientists can analyze this sensor data to identify patterns that indicate impending equipment failure. They build machine-learning models that learn the historical sensor data associated with equipment failures. The models can then scan incoming sensor data and predict future failures.
Reducing Costs and Increasing Uptime
Predictive maintenance reduces costs by eliminating unnecessary routine maintenance and preventing unplanned downtime from equipment failures. Healthcare manufacturers experience less disruption to operations and higher equipment uptime. They can order replacement parts in advance and schedule technicians when machine failures are predicted.
Improving Patient Safety
For healthcare manufacturers, predictive maintenance has significant implications for patient safety. Unplanned equipment downtime can delay or halt the production of critical medical products. Predictive models help manufacturers take a proactive approach to maintenance, ensuring medical devices and equipment are functioning properly to avoid risks to patients who depend on these products.
In summary, predictive maintenance driven by data science allows healthcare manufacturers to optimize equipment performance, reduce operational costs, and uphold high standards for patient safety. By leveraging sensor data and machine learning, healthcare manufacturers can keep medical equipment running efficiently and gain valuable insights into improving maintenance strategies over time. Predictive maintenance is a prime example of how data-driven techniques can transform processes in healthcare manufacturing.
Use Case 2: Quality Control and Assurance
Quality control and assurance are crucial for healthcare manufacturers to ensure patient safety, optimize processes, and maintain high standards. Leveraging data science, healthcare organizations can identify areas of improvement, detect anomalies, and make data-driven decisions by:
Monitoring Product Quality
Healthcare manufacturers can use statistical process control charts, a data visualization method, to monitor quality metrics of products in real-time. By tracking key performance indicators like error rates, defect rates, and variability, organizations can identify when a process is going out of control and needs adjustment. This allows healthcare manufacturers to make data-driven decisions to optimize processes and improve quality.
Detect Anomalies
Healthcare manufacturers generate massive amounts of data across the supply chain, from sourcing raw materials to final packaging. By applying anomaly detection algorithms to this data, organizations can automatically detect irregularities that may impact quality. For example, a sudden change in a manufacturing environment’s temperature or humidity could signal a need for inspection. Catching such anomalies early on helps minimize risks to patients and avoid costly quality issues.
Analyze Quality Trends
Data science techniques like time series analysis allow healthcare manufacturers to identify both seasonal and long-term trends in quality metrics. Recognizing these trends helps organizations determine where to focus improvement efforts and predict future quality performance. For example, an upward trend in product complaints over several months may indicate a need to re-evaluate certain manufacturing processes or raw material suppliers. Identifying such trends proactively can help healthcare manufacturers take corrective actions to ensure quality and patient safety.
Healthcare organizations can leverage data science across the supply chain to monitor quality in real-time, detect anomalies proactively, analyze trends, and make data-driven decisions to optimize processes while ensuring high standards of quality. By taking a proactive approach to quality control and assurance, healthcare manufacturers can achieve operational excellence and provide the best care for patients.
Use Case 3: Supply Chain Optimization
Optimizing the supply chain is crucial for healthcare manufacturers to improve operational efficiency and cost-effectiveness. By leveraging data science, companies can gain valuable insights such as:
Managing Supplier Relationships
Data analysis provides visibility into supplier performance, costs, and risks. Healthcare manufacturers can use data to evaluate suppliers, renegotiate contracts, and identify alternative sources. Monitoring supplier KPIs and compliance data also helps to mitigate risks like production delays, quality issues, and ethical concerns. Optimizing the supplier base and supply chain helps companies reduce costs, improve quality, and build resilience.
Enhancing Distribution Networks
Advanced analytics combined with optimization techniques can help determine the most efficient distribution network design. Factors like production locations, warehouse placement, transportation modes, and delivery routes can be optimized to minimize costs and maximize service. An optimized distribution network allows healthcare manufacturers to get products to customers and patients as quickly and affordably as possible.
By leveraging data science to gain insights into demand, suppliers, and distribution, healthcare manufacturers can make significant improvements in supply chain performance. Continually optimizing operations and the flow of materials and information ultimately helps companies lower costs, reduce waste, and better serve healthcare providers and patients.
Use Case 4: Demand Forecasting
Demand forecasting is essential for healthcare manufacturers to anticipate future needs and optimize production planning by:
Analyzing Historical Demand Patterns
Healthcare manufacturers should analyze historical demand patterns to identify trends, seasonal fluctuations, and other variables that influence demand. Examining several years of historical data on product orders, shipments, and other metrics can uncover recurring patterns to incorporate into demand forecasts.
Factor in External Drivers
In addition to historical data, healthcare manufacturers must consider external drivers that affect demand such as disease outbreaks, medical innovations, policy changes, and more. Monitoring healthcare trends and advancements allows companies to anticipate how these external factors might impact future demand for their products. Data science techniques like sentiment analysis can also provide insights into how public opinion might influence demand.
Develop Statistical Models
Based on their analysis, healthcare manufacturers can develop statistical models to forecast demand. Regression models, time series models, and machine learning algorithms can all be applied to generate predictions. Comparing multiple modeling approaches and combining their results often yields the most accurate forecasts. Models should be tested and validated before being used for production planning.
Continuously Optimize
As new data becomes available, demand forecasts and the underlying models powering them must be re-evaluated. Healthcare manufacturers should continuously monitor how well their forecasts match actual demand and adjust to improve accuracy over time. Comparing forecast errors, updating models, and re-training algorithms with the latest data are all part of optimizing demand forecasting as an ongoing process.
With a data-driven approach to demand forecasting, healthcare manufacturers can gain a competitive advantage through optimized production, ensured availability of products, and the ability to preemptively address changes in the healthcare market. Implementing demand forecasting solutions powered by data science leads to increased efficiency, cost savings, and a better customer experience.
Use Case 5: Personalized Medicine and Drug Development
Personalized medicine and targeted drug development are critical to improving patient outcomes. With the application of data science, healthcare manufacturers can better understand the progression and treatment of diseases through:
Patient Data Aggregation
Healthcare organizations collect massive amounts of data from electronic health records, diagnostic tests, wearable devices, and more. Healthcare manufacturers can tap into these rich data sources through partnerships and collaborations. Aggregating data from disparate sources provides a comprehensive view of a patient’s health background, current conditions, and potential risks.
Insight Discovery
Sophisticated data mining techniques uncover patterns and insights that would otherwise remain hidden. For example, machine learning algorithms can detect segments of patients with similar characteristics and responses to certain drugs. These insights enable healthcare manufacturers to develop treatments targeted to specific patient groups.
Targeted Drug Development
Armed with data-driven insights into disease mechanisms and patient subpopulations, healthcare manufacturers can develop drugs tailored to patients’ unique biological profiles. Targeted drugs may have higher efficacy and fewer side effects compared to one-size-fits-all approaches. Data science accelerates the drug discovery process through predictive modeling and simulation.
Continuous Optimization
Even after a drug has been launched, data collection and analysis continue. Real-world data on treatment responses and outcomes help optimize dosing, identify new uses, and make other improvements. Continuous data science ensures patients receive the maximum benefit from the latest medical innovations.
Use Case 6: Regulatory Compliance and Reporting
To operate legally and ethically, healthcare manufacturers must comply with regulations set by agencies like the Food and Drug Administration (FDA) and report on key performance indicators. Data science facilitates proactive compliance with regulations and comprehensive reporting.
Regulatory compliance refers to the mandatory rules and guidelines that healthcare manufacturers must follow to legally operate. These regulations aim to ensure patient safety, high quality, and ethical practices. Data science enables manufacturers to systematically track compliance with regulations like Good Manufacturing Practices (GMPs) and report on compliance to regulatory bodies. By analyzing data from across operations, manufacturers gain visibility into compliance levels and can address issues to avoid penalties or product recalls.
Performance reporting provides visibility into how well an organization is meeting key objectives and targets. Healthcare manufacturers report on metrics like product quality, customer satisfaction, and sustainability to stakeholders like investors, executives, and regulatory agencies. Data science allows manufacturers to gather data from various sources, analyze trends, and generate reports on key performance indicators (KPIs) to support data-driven decision-making and demonstrate accountability.
In summary, data science is instrumental in managing regulatory compliance and reporting in the healthcare manufacturing sector. By leveraging data analytics and business intelligence, manufacturers can ensure adherence to regulations, monitor compliance levels, and performance reports, and make improvements to optimize processes. Regulatory bodies and stakeholders rely on this data to evaluate manufacturers, hold them accountable, and make policy or investment decisions. Data-driven compliance and reporting are increasingly critical for operational excellence and building trust in the healthcare system.
Use Case 7: Equipment Optimization
Optimizing equipment is crucial for maximizing efficiency and productivity in healthcare manufacturing. By applying data science, healthcare manufacturers can gain valuable insights into equipment performance and identify opportunities for improvement.
One approach is to use predictive maintenance, which leverages sensor data and machine learning to determine the optimal maintenance schedule for equipment. By monitoring equipment conditions in real-time, manufacturers can schedule maintenance right before parts are likely to fail rather than relying on routine maintenance. This reduces unplanned downtime and maximizes the lifetime of equipment.
Another opportunity is using prescriptive analytics to optimize equipment settings and parameters. Collecting and analyzing data on inputs, outputs, and environmental factors helps determine the optimal configuration to maximize metrics like throughput, yield, and quality. These optimized settings can then be automatically implemented to achieve target KPIs.
Data science also enables condition-based monitoring of equipment. Metrics such as vibration, temperature, and pressure are monitored by sensors and analyzed to detect anomalies. Manufacturers are alerted to issues immediately and can take corrective action before there is an impact on production. By catching issues early, unplanned downtime and waste are minimized.
To optimize equipment, healthcare manufacturers should integrate equipment data from sources such as sensors, control systems, and maintenance records. Advanced analytics, machine learning, and data visualization tools can then uncover key insights to enhance planning, maximize performance, reduce costs, and improve productivity. By tapping into data science, healthcare manufacturers gain a competitive advantage through optimized, data-driven equipment management.
Use Case 8: Patient Monitoring and Outcomes Prediction
Data science is enabling new capabilities for patient monitoring and outcome prediction in healthcare manufacturing. By leveraging data from connected medical devices, electronic health records, and other digital health technologies, healthcare organizations can gain real-time visibility into patient health and anticipate potential risks.
Patient monitoring systems that incorporate data science utilize machine learning algorithms to detect anomalies in vital signs and other health metrics. These systems can alert physicians to changes that may indicate a worsening condition so they can intervene quickly. Predictive models can also help determine a patient’s risk of developing a chronic disease or requiring hospital readmission.
Healthcare manufacturers are developing “smart” medical devices with built-in data science capabilities. Connected devices like glucose monitors, blood pressure cuffs, and ECG monitors can analyze a patient’s data for concerning patterns and trigger alerts to prompt medical review. Some devices provide personalized insights and recommendations to help patients better manage health conditions at home.
By leveraging longitudinal data from many patients, healthcare organizations can build predictive models to anticipate outcomes, complications, and costs. Predicting patient mortality, disease progression, and response to treatment can help clinicians make better-informed care decisions. Predicting utilization and spending can also help healthcare organizations allocate resources more efficiently.
Advances in data science have significant implications for patient monitoring and outcome prediction. With connected devices and predictive analytics, healthcare manufacturers and providers can move from reactive to proactive patient care. The future is one of personalized, data-driven medicine where health risks are identified earlier, and adverse events are avoided before they happen. Overall, data science is enabling a smarter, more coordinated healthcare system focused on optimizing patient outcomes.
Use Case 9: Cost Reduction and Efficiency Improvement
To remain competitive, healthcare manufacturers must continually optimize costs and improve operational efficiency. Data science enables organizations to analyze historical data, identify cost drivers, reduce waste, and enhance productivity.
Healthcare manufacturers can leverage data science techniques like predictive modeling to forecast demand more accurately. By predicting product demand, organizations can optimize inventory levels, reduce excess stock, and decrease carrying costs. Data-driven demand planning also allows for more efficient workforce and production scheduling.
Data analysis helps identify areas of waste and inefficiency in the supply chain and manufacturing processes. Organizations can detect bottlenecks, evaluate supplier performance, and redesign workflows to minimize unnecessary steps. Streamlining operations and automating manual tasks boost productivity and reduce labor expenses.
Healthcare manufacturers use data science to perform root cause analysis of quality issues, scrap, and rework. Detecting the sources of defects and failures enables organizations to implement corrective actions, tighten controls, and improve quality. Enhanced quality control results in lower costs from wasted materials, rework, and customer returns.
Leveraging data science, healthcare manufacturers can lower costs through energy efficiency initiatives. Analyzing energy usage patterns helps identify opportunities for conservation and optimization. Organizations can then implement measures like improving insulation, upgrading to energy-efficient equipment, and adjusting thermostat settings during unoccupied periods.
Use Case 10: Risk Management and Mitigation
Risk management and mitigation are crucial for healthcare manufacturers to systematically address risks that could negatively impact their business. By leveraging data science, healthcare manufacturers can gain valuable insights into potential risks and take appropriate actions.
Data science techniques like predictive modeling can help identify risks associated with medical devices or pharmaceutical products in the design and development stages. Detecting risks early on allows healthcare manufacturers to make changes to mitigate issues before products reach the market. Data science also enables healthcare manufacturers to uncover hidden risks in existing products by analyzing large volumes of data from multiple sources such as customer complaints, warranty claims, and safety reports.
Furthermore, healthcare manufacturers can leverage data science for risk monitoring and management. Continuous analysis of risk indicators derived from various data sources allows companies to monitor risks, determine their severity, and take corrective actions as needed. Data science models can also help predict the likelihood and impact of risks, supporting better decision-making.
Risk management is an ongoing process. Healthcare manufacturers must consistently collect data, re-evaluate risks, and make improvements to their risk management strategies. Data science is essential for enabling a proactive and evidence-based approach to risk management. By gaining data-driven insights into risks, healthcare manufacturers can work to avoid or minimize the impact of issues, ultimately enhancing patient safety and the quality of their products.
Conclusion
As we have explored, data science is revolutionizing healthcare manufacturing in myriad ways. From enhancing operational efficiency to predicting outcomes, data-driven insights empower organizations to transform processes, support decision-making, and drive innovation. While the applications highlighted here showcase the incredible potential, they only scratch the surface of what is possible. Data science will continue opening new doors as healthcare manufacturers embrace analytics and leverage the value of data.
You now have a solid foundation to build upon and take the next steps on your data journey. Keep pushing boundaries, ask questions, test hypotheses, and let data be your guide towards unlocking new opportunities and overcoming challenges. The future is bright for healthcare manufacturing organizations that embrace data science as a strategic priority.
Subscribe to my LinkedIn newsletter for exclusive insights on how data science is revolutionizing healthcare manufacturing. Stay ahead of the curve with the latest advancements, case studies, and expert analyses. Don’t miss out – join today to unlock new opportunities in this dynamic industry.
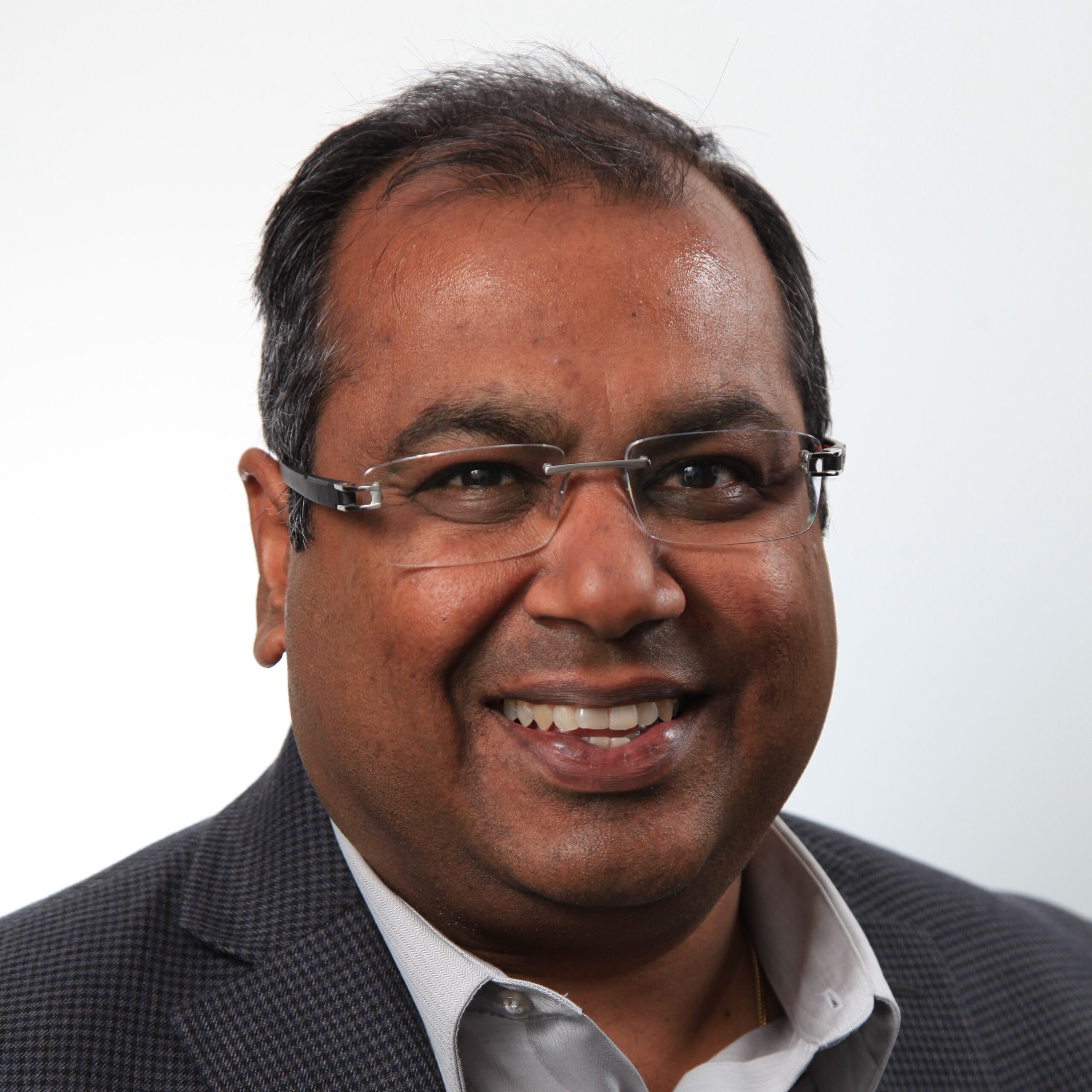
Empowering Healthcare & Smart Manufacturing CXOs | Transforming Challenges into Growth Opportunities with Data-Driven AI Innovation | Microsoft Gold Partner with 30+ years in Data and AI Strategy | #Inc5000 Honoree