From enhancing operational efficiencies to enabling personalized treatments, Data, and AI are transforming healthcare manufacturing in profound ways. As these advanced technologies continue to unlock greater insights from the wealth of data throughout the industry, they present game-changing opportunities to drive better patient outcomes, accelerate innovation cycles, and gain competitive advantages.
This article explores how Data and AI can be strategically leveraged in healthcare manufacturing to extract more value across the product lifecycle – from Research & Development to Sales. We examine specific use cases where manufacturers are already achieving step-changes in productivity, quality, and sustainability through data-driven intelligence.
Read on to understand how you can harness these technologies to enhance decision-making, strengthen collaboration, and create next-generation solutions that tangibly improve patient lives.
Data Explosion in Healthcare Manufacturing
The amount of data collected in the healthcare industry has exploded in recent years. With advancements in electronic health records, medical devices, wearables, and other technologies, the volume of data generated by healthcare manufacturers and providers has reached unprecedented levels. This wealth of data allows healthcare companies to gain valuable insights to improve efficiency, and outcomes and fuel innovation.
Harnessing Data
Data refers to extremely large data sets that may be analyzed computationally to reveal patterns, trends, and associations. In healthcare manufacturing, Data may originate from sources such as:
·      Connected medical devices and equipment.
·      Electronic health records containing patient demographics, diagnoses, medications, treatment plans, and outcomes
·      Genomic and biological data from research and clinical trials
·      Data from wearable technologies and mobile health apps
By aggregating and analyzing these diverse data sets using data mining techniques, key insights can emerge to optimize manufacturing processes, reduce waste, predict future equipment needs, and support product development. Identifying trends across huge populations can also help manufacturers gain a more comprehensive view of the real-world impacts of their products and services.
The Rise of AI and Machine Learning
Artificial intelligence and machine learning are enabling healthcare manufacturers to analyze Data in new ways. AI systems can detect complex patterns in massive amounts of data that humans alone may miss. Machine learning algorithms can then use those patterns to make predictions or recommendations to help guide decision-making. AI and ML have the potential to transform areas such as:
Predictive maintenance: Analyzing data from connected medical devices and equipment to anticipate when parts may fail and proactively schedule service.
Personalized product design: Gaining insights into different patient populations and needs to develop customized medical products tailored to specific groups.
Automated quality control: Detecting anomalies in manufacturing processes faster to ensure high-quality standards are met.
Enhanced diagnostics and treatment: Identifying patterns in patient data and outcomes to support the development of more accurate diagnostic tools and optimized treatment plans.
With the increasing availability of healthcare data and rapid progress in AI, healthcare manufacturers are poised to gain valuable insights that can drive significant improvements in efficiency, outcomes, and innovation. Effectively leveraging Data and AI will be key to success in the era of data-driven healthcare.
How Data Is Transforming the Industry
Data refers to extremely large data sets that may be analyzed computationally to reveal patterns, trends, and associations. In healthcare manufacturing, Data enables companies to gain valuable insights to optimize processes, reduce costs, and improve outcomes.
Optimizing Efficiency and Quality
By analyzing massive volumes of data from sensors, equipment, and operations, manufacturers can identify inefficiencies and inconsistencies. They can pinpoint bottlenecks, reduce variability, and enhance productivity. Data also allows constant monitoring of quality metrics to minimize defects and ensure the highest standards are met.
Personalizing Products and Treatments
Aggregating and analyzing patient data, treatment outcomes, and other health metrics allows companies to develop solutions tailored to specific patient groups or even individuals. They can gain insights into how patients respond to different therapies and customize products and services accordingly. This data-driven personalization of healthcare helps improve patient experiences and outcomes.
Driving Innovation
Examining huge datasets aggregated from various sources exposes connections that would otherwise remain hidden. These types of correlations and insights fuel discoveries and spur innovative ideas. Data analytics provides the foundation for groundbreaking advancements by revealing patterns that could lead to new products, more effective treatments, and even cures for complex conditions.
Implementing predictive analytics
By applying machine learning and artificial intelligence to massive datasets, healthcare manufacturing companies can develop predictive models to anticipate future outcomes, events, or behaviors. Predictive analytics allows them to forecast things like resource demand, treatment efficacy for different patients, and risks of complications—all to drive better decision-making and planning.
Improving Efficiency and Productivity with Data Analytics
Data and AI provide powerful tools for optimizing efficiency and productivity in healthcare manufacturing. By analyzing large datasets, identifying patterns, and applying machine learning algorithms, companies can uncover actionable insights to streamline processes, reduce waste, and improve outcomes.
Optimizing Operations with Predictive Analytics
Predictive analytics leverage historical data to model future events or behaviors. Manufacturers can use predictive modeling to anticipate demand, machine failures, or supply chain disruptions. By predicting likely scenarios, companies can optimize operations and resource allocation. For example, predicting higher demand for a product allows increasing production to avoid stockouts while predicting a machine failure allows proactively scheduling maintenance to minimize downtime.
Automating Repetitive Tasks
Many manufacturing processes involve repetitive, routine tasks that can be automated using robotics and AI. Automation speeds up processes, reduces human error, and allows employees to focus on higher-level work. For example, AI-powered robotic arms and conveyor belts are used extensively in pharmaceutical manufacturing to handle repetitive jobs like moving, sorting, and packaging products.
Improving Quality Control
Advanced image recognition software can automatically detect anomalies or defects in products. The AI analyzes high-resolution images to identify issues that may escape human inspectors. For example, AI systems are used to scan medical devices, pill imprints, or the fill levels of injectable drugs to ensure conformity to strict quality standards. Any detected issues can be flagged for further review to avoid compromising patient safety or product quality.
Optimizing Supply Chains with Predictive Analytics
To improve efficiency and outcomes, healthcare manufacturers can leverage predictive analytics to optimize their supply chains. By analyzing historical data and patterns, predictive models can forecast future demand, enabling proactive planning and risk mitigation.
Forecasting Demand
Predictive analytics use machine learning algorithms to analyze factors like past sales, trends, seasonal patterns, and external events to predict future demand for products. Accurately forecasting demand allows manufacturers to optimize inventory levels, ensuring adequate supply without excess stock. This reduces waste and frees up working capital.
Managing Risks
Predictive analytics also help identify potential risks to the supply chain like production disruptions, transportation issues, or raw material shortages. By simulating different scenarios, manufacturers can assess the impact of risks and determine appropriate mitigation strategies before issues arise. This proactive risk management improves supply chain resilience and continuity.
Optimizing Operations
With demand forecasts and risk assessments, manufacturers can make data-driven decisions to optimize supply chain operations. This includes synchronizing production schedules, optimizing transportation routes and warehousing, and determining safety stock levels. Optimized operations enable substantial cost savings through improved efficiency, reduced waste, and maximized throughput.
Personalizing Treatments with Data-Driven Insights
In the realm of healthcare manufacturing, the ability to personalize treatments based on individual patient needs is a transformative advancement made possible by Data and AI-driven insights. By harnessing vast amounts of data from electronic health records, genomic information, wearable devices, and other sources, manufacturers can gain a deeper understanding of patient populations and their responses to various therapies. This knowledge allows for the development of tailored treatments that consider factors such as genetic predispositions, lifestyle habits, and environmental influences. Here’s how personalized treatments are revolutionizing healthcare manufacturing:
Precision Medicine: Data analytics enable the identification of biomarkers and genetic markers associated with specific diseases or conditions. By analyzing this information alongside patient data, manufacturers can develop targeted therapies that address the underlying molecular mechanisms driving disease progression. Precision medicine improves treatment efficacy and minimizes adverse effects by ensuring interventions are tailored to each patient’s unique biology.
Real-Time Monitoring and Adjustment: Connected medical devices and wearables provide continuous streams of health data, allowing for real-time monitoring of patient health metrics. AI algorithms can analyze this data to detect subtle changes indicative of disease progression or treatment response. Manufacturers can then use these insights to adjust treatment regimens in real time, optimizing outcomes and minimizing the risk of complications.
Predictive Modeling for Treatment Outcomes: Machine learning models can analyze historical patient data to predict the likely outcomes of different treatment strategies. By identifying patterns in patient responses, manufacturers can anticipate which treatments are most likely to be effective for individual patients. This predictive modeling enhances clinical decision-making, enabling healthcare providers to choose the most appropriate interventions for each patient.
Enhanced Patient Engagement: Personalized treatments empower patients to take an active role in managing their health. By providing patients with personalized treatment plans based on their unique characteristics and preferences, manufacturers can improve treatment adherence and patient satisfaction. AI-powered tools, such as virtual health assistants, can deliver personalized education and support to patients, helping them better understand their conditions and treatment options.
Continuous Learning and Improvement: As more data becomes available and AI algorithms continue to evolve, personalized treatments will become increasingly refined and effective over time. Manufacturers can leverage feedback from real-world treatment outcomes to continuously refine treatment algorithms and improve patient outcomes. This iterative process of learning and improvement represents a paradigm shift in healthcare manufacturing, moving towards truly personalized and data-driven care.
The Future of AI-Powered Research & Development in Pharmaceutical Industry
The pharmaceutical industry is undergoing a profound transformation driven by advancements in AI and Data analytics. These technologies are revolutionizing the drug discovery and development process, enabling manufacturers to bring new therapies to market faster, more efficiently, and with greater precision than ever before. Here’s how AI is reshaping the future of pharma R&D:
Accelerated Drug Discovery: Traditional drug discovery methods are time-consuming and costly, often taking years to identify potential drug candidates. AI-powered algorithms can rapidly analyze vast amounts of biomedical data, including genomic information, protein structures, and disease pathways, to identify promising drug targets and compounds. By streamlining the drug discovery process, AI enables manufacturers to identify potential therapies more quickly and cost-effectively.
Virtual Screening and Molecular Design: AI algorithms can predict the biological activity and safety profiles of drug candidates, allowing manufacturers to prioritize compounds with the highest likelihood of success. Virtual screening techniques leverage machine learning models to sift through large chemical libraries and identify molecules that are likely to bind to a target protein with high affinity. Similarly, AI-driven molecular design tools can generate novel drug candidates with optimized pharmacokinetic properties and reduced off-target effects.
Predictive Modeling for Clinical Trials: AI algorithms can analyze patient data from electronic health records, clinical trials, and real-world evidence to predict patient responses to experimental therapies. By identifying patient subgroups that are most likely to benefit from a particular treatment, manufacturers can design more targeted and efficient clinical trials. Predictive modeling also enables the identification of potential safety issues earlier in the drug development process, reducing the risk of adverse events during clinical testing.
Drug Repurposing and Combination Therapies: AI algorithms can analyze vast amounts of biomedical data to identify new uses for existing drugs and potential synergistic combinations of therapeutics. Drug repurposing, or repositioning, involves identifying novel indications for approved drugs based on their known pharmacological properties. Similarly, AI-driven combination therapy approaches leverage synergistic interactions between different drugs to enhance efficacy and reduce the risk of drug resistance.
In summary, AI set to accelerate the drug discovery and development process, enabling the development of personalized therapies, and improving the efficiency and success rate of clinical trials. By using AI and Data analytics, manufacturers can bring new therapies to market faster, more efficiently, and with greater precision, ultimately benefiting patients and society.
Overcoming Challenges in Implementing Data and AI
While Data and AI offer tremendous potential to transform healthcare manufacturing, implementing these technologies presents a unique set of challenges. From data privacy concerns to technical limitations, overcoming these obstacles is essential to realizing the full benefits of Data and AI in the industry. Here are some key challenges and strategies for overcoming them:
Data Quality and Accessibility: One of the primary challenges in leveraging Data is ensuring data quality and accessibility. Healthcare data is often fragmented, incomplete, and stored in disparate systems, making it challenging to aggregate and analyze effectively. Manufacturers must invest in data integration and standardization efforts to ensure that data is accurate, reliable, and accessible across the organization.
Data Privacy and Security: Healthcare data is highly sensitive and subject to strict privacy regulations, such as HIPAA in the United States and GDPR in the European Union. Manufacturers must implement robust data security measures to protect patient privacy and comply with regulatory requirements. This includes encryption, access controls, and data anonymization techniques to prevent unauthorized access or disclosure of sensitive information.
Lack of Technical Expertise: Implementing AI and Data solutions requires specialized technical expertise in areas such as data science, machine learning, and software development. Manufacturers may face challenges in recruiting and retaining qualified professionals with the necessary skills and experience. Investing in training and development programs can help build internal expertise and capacity to support AI and Data initiatives.
Ethical and Regulatory Considerations: AI algorithms can inadvertently perpetuate bias or discrimination if trained on biased data or flawed assumptions. Manufacturers must ensure that AI systems are developed and deployed ethically and responsibly, taking steps to mitigate bias and promote fairness and transparency. Additionally, manufacturers must navigate complex regulatory frameworks governing the use of AI and Data in healthcare to ensure compliance with applicable laws and regulations.
Cost and ROI Concerns: Implementing AI and Data solutions requires significant upfront investment in infrastructure, technology, and talent. Manufacturers may face challenges in demonstrating the return on investment (ROI) of these initiatives, particularly in the early stages of implementation. To overcome this challenge, manufacturers should develop clear business cases and metrics for success, focusing on tangible outcomes such as improved efficiency, productivity, and patient outcomes.
FAQs on Data and AI in Healthcare Manufacturing
What is Data, and how is it used in healthcare manufacturing?
Data refers to extremely large datasets that can be analyzed computationally to reveal patterns, trends, and associations. In healthcare manufacturing, Data is used to optimize processes, reduce costs, improve outcomes, and drive innovation. Manufacturers analyze data from sources such as electronic health records, medical devices, wearables, and research studies to gain insights into patient populations, treatment efficacy, and manufacturing processes.
What is artificial intelligence, and how is it transforming healthcare manufacturing?
Artificial intelligence (AI) refers to the simulation of human intelligence in machines, enabling them to perform tasks that typically require human intelligence, such as perception, reasoning, learning, and decision-making. In healthcare manufacturing, AI is transforming processes such as predictive maintenance, personalized product design, automated quality control, and enhanced diagnostics and treatment. AI algorithms analyze Data to detect patterns, make predictions, and optimize outcomes, leading to greater efficiency, productivity, and innovation.
What are some specific use cases of Data and AI in healthcare manufacturing?
Some specific use cases of Data and AI in healthcare manufacturing include predictive maintenance of medical equipment, personalized product design based on patient data, automated quality control in manufacturing processes, and enhanced diagnostics and treatment planning using AI algorithms. These technologies enable manufacturers to optimize efficiency, improve quality, and develop innovative solutions that positively impact patient outcomes and experiences.
What are the challenges of implementing Data and AI in healthcare manufacturing?
Implementing Data and AI in healthcare manufacturing presents challenges related to data quality, privacy, technical expertise, ethics, and cost. Manufacturers must ensure that data is accurate, reliable, and accessible while protecting patient privacy and complying with regulatory requirements. They also need to recruit and retain qualified professionals with the necessary skills and experience to develop and deploy AI solutions. Additionally, manufacturers must address ethical considerations related to bias, fairness, and transparency and demonstrate the ROI of Data and AI initiatives.
What are the benefits of leveraging Data and AI in healthcare manufacturing?
Leveraging Data and AI offers numerous benefits for healthcare manufacturing, including improved efficiency, productivity, quality, and innovation. Manufacturers can optimize processes, reduce costs, minimize errors, and develop personalized treatments tailored to individual patient needs. These technologies enable manufacturers to gain actionable insights from data, make data-driven decisions, and continuously improve outcomes and experiences for both patients and healthcare providers.
Conclusion
Data and AI are revolutionizing healthcare manufacturing, enabling companies to extract valuable insights from data, optimize processes, and develop innovative solutions that improve patient outcomes and experiences. By leveraging Data and AI, manufacturers can personalize treatments, accelerate drug discovery and development, overcome challenges, and drive innovation in the industry. While implementing these technologies presents challenges, the potential benefits are immense, paving the way for a future of data-driven healthcare manufacturing that prioritizes efficiency, quality, and patient-centric care. As manufacturers continue to harness the power of Data and AI, the possibilities for transformative change are limitless, offering hope for a healthier, more sustainable future for all.
Subscribe to my LinkedIn Newsletter for the latest insights on how Data and AI are transforming healthcare manufacturing. Stay updated on personalized treatments, accelerated drug discovery, and more. Join the conversation for a healthier, more sustainable future. Subscribe now!
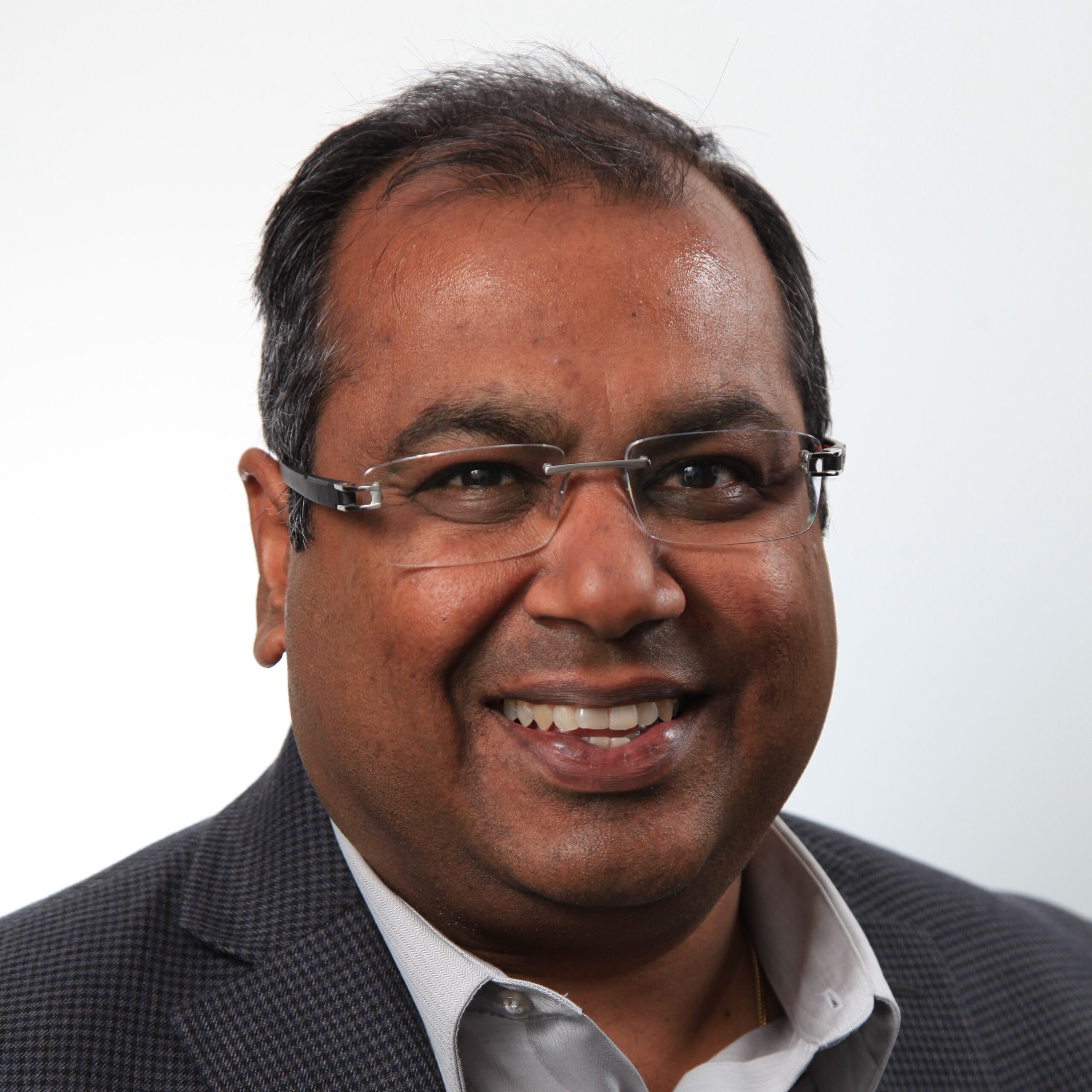
Empowering Healthcare & Smart Manufacturing CXOs | Transforming Challenges into Growth Opportunities with Data-Driven AI Innovation | Microsoft Gold Partner with 30+ years in Data and AI Strategy | #Inc5000 Honoree